Francesco Sguanci
1 Year Ago
One to One Messaging
It could be a title of a movie: “Mission Impossible: Getting the right message to the right people at the right time.” Marketers understand the importance of strategic targeted messaging, and while there still are a lot of companies that practice the “one-size-fits-all” approach, others have started to embrace propensity modeling to create better personalization and achieve higher ROI.
This application of mathematical models to predict whether someone will take a particular action is a powerful way to identify whom among your audience is most likely to engage in a particular behavior, and under what circumstances. With the aid of propensity modeling, you can focus your resources on the people for whom engagement will generate a meaningful change in behavior, giving them the nudge they need to pull the trigger.
Can We Do “One to One” Targeting in Debt Collection?
Given that propensity modelling is used to generate some desired change in behavior, why should we restrict its application only to marketing activities? Other industries operating outside of the world of E-Commerce and Retail can benefit greatly from propensity modeling. The banking industry, for example, can greatly benefit from the implementation of propensity modeling for debt collection efforts – otherwise known as “propensity to pay”. While banks often have good processes in place for collecting debt, they often lack the insight to decide which processes should be applied to which customers.
Let’s expand on this concept through the aid of a real case study from one of Dunn Solutions’ financial clients.
In 2018, a major financial institution in the Midwest commissioned a propensity to pay model to be applied to delinquent accounts (customers who are late in paying their outstanding balance). They were familiar with the concept of “scoring” customers; however, an earlier attempt at developing an in-house solution had failed to deliver the Expected Return on Investment.
This came as no surprise. In fact, most companies are not successful with propensity modelling because they don’t act on their propensity scores in the correct way. For example, a company might be tempted to target only the delinquent accounts with the highest propensity to pay. As logical as it may seem, this overlooks the fact that the majority of delinquent accounts will “self-cure.” This means these accounts might become delinquent simply due to an overlooked due date, but will immediately course-correct and pay when they realize the error. These customers might have a high propensity score; however, because they will “self-cure,” companies are wasting money and resources by contacting them. In other words: these customers have a high ability to pay, and will do so without the debt collection efforts of the company.
This was exactly the scenario in which our client found themselves; they were not finding success with their propensity modeling .
Dunn Solutions Delivers a Successful Propensity to Pay Model
Dunn Solutions created an accurate and scalable propensity-to-pay model, and developed a soup-to-nuts comprehensive strategy for using its outputs.
To start: Dunn Solutions conducted a full-data-evaluation in order to understand what variables could be used and how the population of interest should be defined. Based on the outstanding balance of the population of interest, we formulated a “what-if” analysis under different scenarios to understand the possible ROI from the project – for example: if we could increase debt collection by 5%, 10%, or 15%, what would our return on investment be?
Next, along with the client’s IT team, we designed an efficient data pipeline for regular data ingestion to score delinquent accounts in near real-time, as well as a feedback loop to retrain the model on a regular basis. Only when these preliminary steps were fully executed, did we move on with the development of the propensity-to-pay model.
The next step in the process was to develop and test competing models, using an unseen sample of delinquent accounts for validation purposes. Once the propensity-to-pay model was validated and the accuracy proven, we developed an implementation strategy, and tested it in a controlled experiment.
We recommended that customers with very high propensity to pay NOT be contacted, as these accounts have the ability to pay and will cure themselves. Finally, customers with extremely low propensity scores should also be ignored, as they are too far gone for debt collection efforts to be successful. Instead, priority was given to the middle-tier customers, who are really at risk of defaulting permanently, but who could still be persuaded with the right incentive.
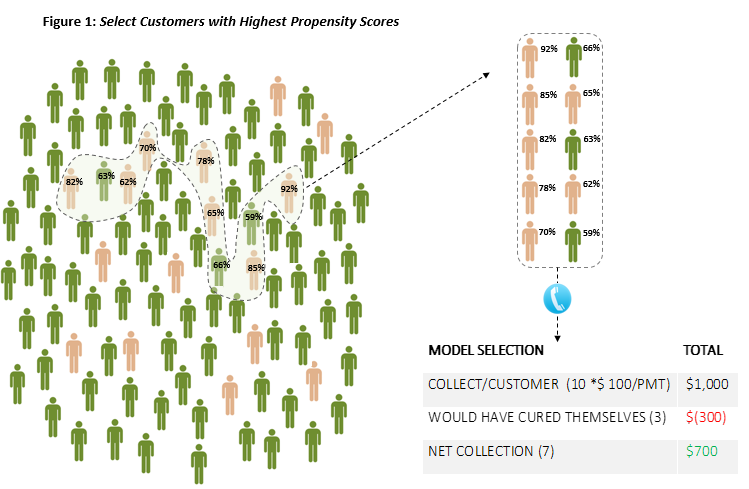
After a few months of market testing using different incentives, these were the expected annualized results:
- 41% improvement in debt collection amounts collected
- 49% decrease in outbound phone calls
- 18% decrease in accounts sent to external legal debt collection agencies
The Bottom Line: While many vendors offer some form of propensity-to-pay modeling solution, the most sophisticated statistical methodology is only as good as its end-to-end solution. Additionally, if the methodology is not part of a continuous feedback loop that adapts to new situations and learns from new data, your ROI will be low.
Here at Dunn Solutions we have demonstrated ROI over many different projects by fully partnering with our clients to achieve state-of-the art and customized solutions to meet their unique needs. Please feel free to contact us with any questions you might have on propensity-to-pay models!