Francesco Sguanci
1 Year Ago
Despite playing a major role in the economy, the business to business (B2B) sector largely lags behind the business-to-consumer (B2C) market in adopting predictive analytics for business decisions, and looking at Dunn Solutions’ clients I can confirm that this is the case. One area of particular interest is pricing; unfortunately, many companies still see pricing in B2B as a domain where soft-skills and interpersonal skills can’t be substituted, fully or partially, by machine learning tools.
The Challenge of B2B Pricing
B2B pricing decisions differ substantially from those within the business-to-consumer (B2C) market because of the high complexity of the business transactions, where the customer needs to make several interrelated decisions, and the salesperson needs to be able to “read” the customer mindset before generating a quote. But, in my experience with Dunn Solutions’ B2B clients, I have found that it is this very complexity that creates a potential for leveraging predictive analytics techniques and machine learning.
Leveraging Predictive Analytics for B2B Pricing
Sellers in B2B contexts can charge their customers different prices for the same products, and often can even change the price from one purchase to another with little notice. Obviously, this kind of “price discrimination” creates plenty of opportunities to increase short-term profit. However, it can negatively impact the development of trust needed to establish a long-term relationship with buyers. For this reason, a good statistical methodology should be able to predict the short-term impact of pricing as well as the long-term effects of pricing decisions.
Dunn Solutions' Solution
Using transactional data from a manufacturer of cleaning supplies, the Dunn Solutions' Data Science Team developed dynamic statistical pricing models that are capable of modeling all the buyer’s interrelated decisions (how much to purchase, whether to request a quote and, if so, whether to accept the seller’s bid) as well as simulating the evolution of the buyer-seller relationship over time.
The exact form of the pricing model needs to be adjusted based on a few factors (whether the business sells commodities or manufactured goods, whether only direct channels vs. resellers are considered, etc.). For the specific client mentioned above, three inter-dependent events were modeled:
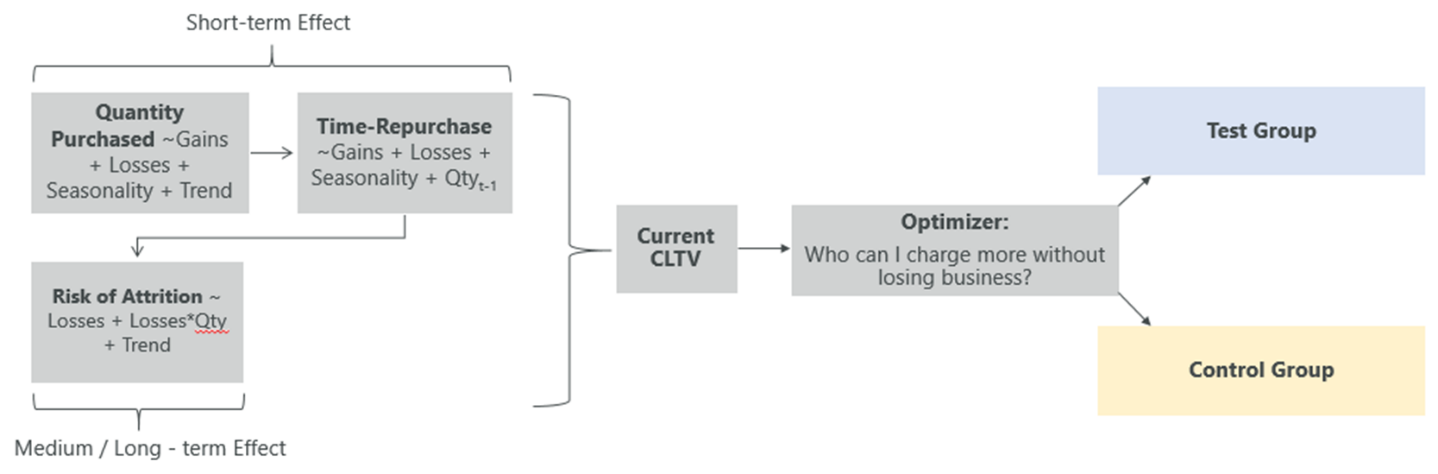
1. The impact of a price increase or decrease on the very next purchase
2. The impact of a price increase or decrease and the quantity purchased on the time between transactions
3. Change in risk of attrition due to price increase or decrease
The Results
Using the derived weights as inputs to our profit-maximizing optimizer, Dunn Solutions was able to create a personalized pricing strategy for each combination of buyer and product that ensured both short term and long-term profitability. In a randomized Test/Control market test, we observed that the $ margins for the Test Group (with optimized prices) were ~11% higher than $ margins for the Control Group (with the unchanged prices) at the end of the 9-month observation period.
The Role of Pricing Modeling
I do not suggest in any way that Pricing Modeling should replace the salesperson; rather, I see it as a tool to help the salesperson generate profits significantly higher than the salesperson could do alone. Creating good pricing models, however, requires significant knowledge and can be challenging. Let Dunn Solutions' Data Science team build you the models that maximize your short term and long-term margins.
Contact Dunn Solutions today to discuss how we can help your company grow!