Does your organization need a data lake, in addition to a data warehouse? Regardless of whether you have a data warehouse or not, the answer is YES: your organization needs a data lake.
Managers and analysts love data warehouses, but Dunn Solutions hears (and experiences) a consistent complaint from data scientists: “the enterprise data warehouse does not meet our data needs!”
The data warehouse is a centralized repository where data is stored that provides one version of the truth. This would certainly be a place where a data scientist could access high quality data. What is it about the warehouse that falls short of their expectations?
Consumers of data within an organization can be divided into three categories:
- Give Me What I Need 80% of data consumers (management and front-line workers) get what they need from an enterprise data warehouse.
- Data Analysts 15% of data consumers (analysts) get information from the data warehouse and transactional reports.
- Data Scientists 5% of data consumers (data scientists) are voracious data consumers, and the data warehouse does not begin to satisfy their hunger!
While data scientists represent the smallest proportion of these groups, they represent the group that provides the biggest impact on the business! Data scientists are responsible for advanced analytics, which is necessary to be competitive. They can use data to help make informed decisions.
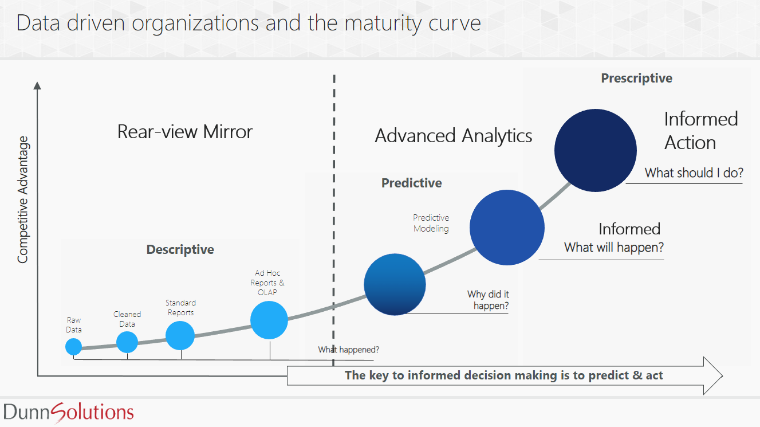
Predictive Analytics Needs a Data Lake
Data warehouses, by their very definition, can never meet the needs of the data scientist. The data warehouse is a vital component of business analytics, and the data warehouse supports key performance indicators and dashboards. And to support this, the data warehouse stores attributes relevant to the business processes being measured. This greatly limits what the data scientist can do (with the information in the data warehouse)!
Data warehouses typically support descriptive analytics. The information is used in dashboards and reports to monitor well-defined business processes. Think, “are my revenues as expected for this time of year?,” “Are any product lines under or over performing year over year?” While all this data in the warehouse is valuable, it leaves the data scientist starving for more variety in attributes.
These business process questions are answered with the data found in a data warehouse. So, what happens with data that does not belong in the data warehouse? It is either lost or lives buried in the various systems used to run the business.
I will just put all my data into the data warehouse, it is a warehouse after all!
There are a lot of data elements that can prove useful for data science, but never make it (or do not belong) in the data warehouse. It takes a lot of effort and money to integrate new data into a data warehouse, so we only include data to help make decisions. Data that supports KPIs (Key Performance Indicators) must go through a formal series of steps to be integrated into the warehouse.
Keep in mind that the amount of data is not the issue. It is the effort in designing and developing the code that populates the data warehouse that is the issue! The effort required to write ETL makes it cost prohibitive to load everything into a data warehouse. And since you do not know what data may contribute to a predictive model you would be expending time and money processing data that may serve no purpose!
Where do Data Scientists go to get their Data?
Data that supports data science should be stored in a data lake. A data lake is a centralized location to store unstructured, semi-structured and structured data. Unlike a data warehouse, a data lake is used to capture as much information as we can regardless of whether we need it today (or not).

For example, if you want to understand what customers are saying about your products in social media, you need to capture that information as it is being created. Then, data scientists can create sentiment analysis models which in turn can be used to monitor sentiment in real time!
Data Scientists Spend 80% of their Time Wrangling Data from Various Sources
Data wrangling from source systems is the thing that slows down a data scientist. If data is not centralized, data scientists must track it down, extract it, and put it somewhere – data could be in transactional databases, events logs, social media, and more!
To accelerate data wrangling, we want to make sure the data is in one central repository – a “playground” for the data scientist. Having a central repository is also good for data governance. As with a warehouse, teams can decide what data goes into a data lake and who can access it. However, since a data lake contains unstructured data, the formal processes to add data into a data lake can be much quicker.
What Is A Data Lake? Does a Data Lake replace a Data Warehouse?
A data lake is more than a playground for the data scientists. The data lake can be used to stage data for your data warehouse. You can keep all your staged data for all time. You can track metrics that may not seem important for the warehouse today but could be easily added to the warehouse in the future. Also, a data lake can be used to rebuild a data warehouse if something happens to it. Developers can transform data on-the-fly when loading data lake information into a data warehouse.
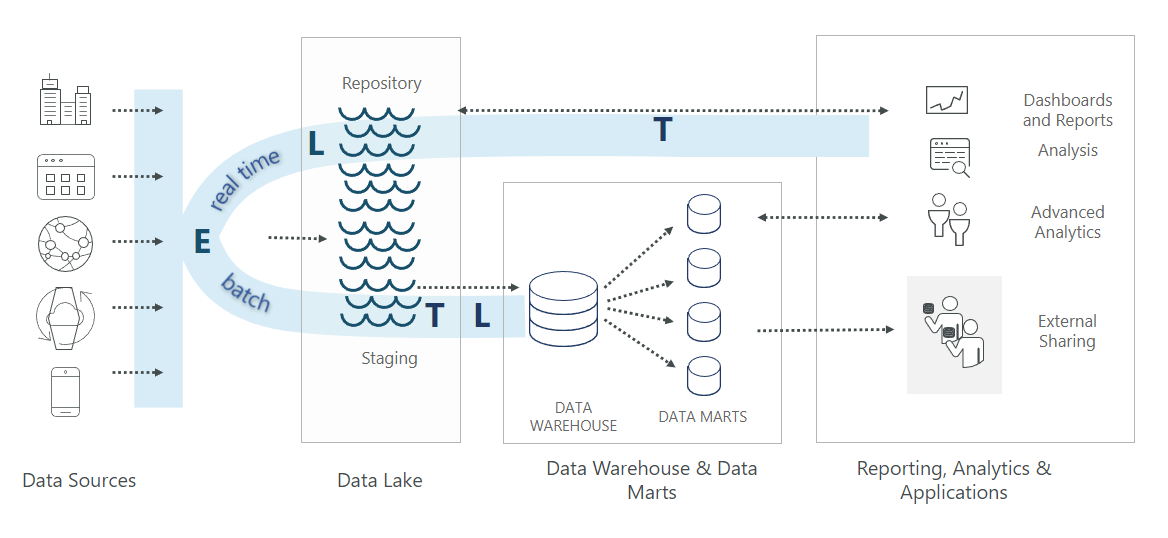
Also, the results from predictive models (that were created from data in a data lake), can be sent to the data warehouse so it can be used in reports and dashboards! Imagine a dashboard that not only shows you how much a customer has spent with you, but also the customer’s lifetime value.
Should my Organization Have a Data Lake?
Data warehouses are data repositories to support reports and dashboards that measure business processes. A data lake supports advanced analytics and data scientists. Advanced analytics is critical for your organization's ability to make better business decisions and improve any business goal (i.e., more revenue, more profit, less defects, focused marketing spend, etc.)
The data lake does not replace the data warehouse— the two work together to meet the needs of the type of data that organizations require. Data warehouses store data for KPIs, metrics, and dashboards used to support business decisions. Data lakes store raw data used for predictive modeling to support future decisions.
A data driven organization needs both data lakes and data warehouses. Does your organization have a data warehouse? Are you considering a data lake? Can you start with a data lake even if you do not have a data warehouse? Dunn Solutions can help you with those questions.
Empower Your Organization with a Data Lake
Dunn Solutions’ data lake experts have executed many Data Lakes projects. Our data lake team will help you capture and store this valuable information in a data lake so that your analysts and research teams have data to find the next big thing, whether it be a trend, anomaly, or actionable insight.
Learn More and Contact Us Today to learn how we can help your business.
Authors: Brandon Novy, Jose Hernandez
Posted: December 21, 2022